After several years of laborious effort, we are proud to announce the public release of the new Deflect software ecosystem. In our Github organization https://github.com/deflect-ca you will find all official Deflect related open source repositories that allow you to stand up an entire website protection infrastructure or its individual components. Deflect offers a high-performance content caching and delivery network, cyber attack mitigation tools powered by Banjax and the Baskerville machine learning clearinghouse, user dashboards, APIs and much else. You are reading this post on a rebuilt and refactored Deflect infrastructure and we’re very proud of that!
Below is the story of the how the new Deflect came to be and rationale for making various software choices.
Deflect-next
Created in 2011 Deflect was a relatively simple solution to the many-against-one problem of distributed denial of service (DDoS) attacks targeting civil society’s web servers. By running Deflect’s caching and mitigation software on constantly rotating edge servers strategically located in some of the world’s biggest data centers, we offered a many-against-many scenario, utilizing the widespread nature of the Internet in a similar manner to those organizing attacks against our clients – leveling the playing field and bringing a little more ‘equalitie’ for our clients’ rights to freedom of expression and association with our audience. Deflect edges memorized previous requests for website data (by virtue of reverse-proxy caching techniques) and removed the load from our clients’ web servers. To block the onslaught of bot-driven attacks, we developed rule based attack mitigation software – Banjax – identifying algorithmic behavior that we considered malicious and banning the IPs that exhibited them.
As we scaled our infrastructure and hundreds of independent media, human rights groups and other non-profits joined the service, millions of daily requests were received on the Deflect network from around the world. This growth was matched by a higher frequency and sophistication of attacks. The Deflect infrastructure was continuously and laboriously patched, improved and upgraded multiple times. As often the case, the software code underpinning the service became cumbersome and replete with complicated configurations and fixes. Moreover, we moved further and further away from our secondary project ambition – to see other technology groups run their own instances of Deflect using our code base. The software stack required a lot of manual configuration and ‘insider knoweledge’. Beginning in 2019 we began to architect and develop a new version of Deflect, using an entirely new method of provisioning, managing and configuring network components, maintaining our agility and adding reproduce-ability as a primary design philosophy.

From ATS to Nginx
The primary tool for serving Deflect clients’ websites is the caching software installed on every network edge. It does all the heavy lifting in our design – fielding requests from the Internet on behalf of our clients’ websites in a reverse proxy fashion. Initially we had opted for the Apache Traffic Server (ATS) built by Yahoo and released open source in early 2000s. The choice was made primarily for its performance levels under stress tests. The software itself was not yet widespread and a little more difficult to set-up and maintain, with configuration for single actions often spread across multiple files. It required every Deflect network operator to dig deep into documentation and source code to figure out what will happen with every change.
Another caching and proxy solution – Nginx – was a more attractive choice for our new network design, with expressive configuration formats and a much larger technical userbase.
From Ansible and Bash to Python and Docker
Deflect clients customize their caching and attack mitigation settings via the Deflect Dashboard, which sends snapshots of these settings to the network controller. Previously, the configuration engine was a mix of Ansible and Bash, and it had overgrown in logic and depth more than what was destined to be managed in these languages. We have now rebuilt the configuration module in Python, supporting better scaling in the future and API communications.
We rebuilt the orchestration module of Deflect – for installing packages, starting and stopping processes, and sending new configuration to network edges, using Docker.
The benefits of containerization are well-known, but in short the advantages to us were: decoupling applications from the host OS (upgrading between Debian versions has historically been a pain point), making our various development and staging environments more reproducible, and letting us create and destroy multiple copies of a container on the same server easily. Once our applications were containerized, we needed a way to start and stop these containers, deciding to write it imperatively in our go-to general-purpose language, Python. There’s a library, docker-py, which connects to the Docker daemon on remote hosts over SSH and provides an API for building images, creating volumes, starting/stopping containers, and everything else we needed. The result is not as simple as Docker Compose or Swarm, but not as complicated as Kubernetes, and is written in a language everyone on our dev team already knows.

From Banjax to Banjax-go
Our primary method of mitigation – Banjax – was previously an ATS plugin, and as such was tightly coupled to the internal details of ATS’s request processing state machine and event loop. Written as C++ code it was tightly coupled with ATS internals and often limited in functionality by the caching server itself. Answering a simple question like “does a request count against a rate limit even if the result has previously been cached, or only if the request goes through to the origin?” required a close reading of Banjax and ATS source code. To port our attack mitigation logic to another server like Nginx would require a similarly detailed understanding of its internals. In addition, we wanted to share Banjax tooling with others – but could not decouple it from ATS – no one from our partners and peers were running this caching software.
We explored an alternate architecture: where attack mitigation logic lives in a separate process (developed in any language, and decoupled from the internals of any specific server) and talks to the server over some inter-process communication channel. We explored using an Nginx plugin which was specialized for this purpose (and developed a proof-of-concept ATS plugin in Lua which did the same thing) but found that a combination between the very widely used `proxy_pass` directive and `X-Accel-Redirect` header was more flexible (authentication responses can redirect the client to arbitrary locations) and probably more portable across servers. As for the choice of language for the authentication service, Python and the Flask framework would have been nice because we use it elsewhere in our stack, but some benchmarks showed Go and Gin being a lot faster (we were aiming for a worst-case overhead of about 1ms on top of requests with a 50th percentile response time of 50ms, and Go/Gin achieves this).
The interface between Nginx and Banjax-go is a good demonstration of Nginx’s expressive configuration file. This first code block says to match on every incoming request (`/`) and proxy the request to `http://banjax-go/auth-request`.
location / { proxy_pass http://banjax-go/auth_request }
Banjax-go then checks the client IP and site name against its lists of IPs to block or challenge. It responds to Nginx with a header that looks like `X-Accel-Redirect: @access_granted` or `X-Accel-Redirect: @access_denied`. These are names of other location blocks in the Nginx configuration, and Nginx performs an internal redirect to one of them.
location @access_granted { proxy_pass https://origin-server } location @access_denied { return 403 "access denied"; }
This is already a lot easier to understand than a plugin which hooks into the internals of Nginx’s or ATS’s request processing logic (reading and writing a configuration file is easier than reading and writing code). Furthermore it composes nicely with the other concepts that can be expressed with Nginx’s scoped configuration: you can control the logging, caching, error-handling and more in each location block and its clear whether it applies to the request to banjax-go, the request to the origin server, or the static 403 access denied message.
Here’s a diagram that shows the above proxy_pass + X-Accel-Redirect flow (follow the red numbers 1, 2, and 3) along with the other interfaces Banjax-go has: the log tailing and the Kafka connection. The log tailing enforces the same regex + rate limit rules that the ATS plugin did, but asynchronously (outside of the client’s request and response) rather than synchronously. The Kafka channel is for receiving decisions from Baskerville (“challenge this IP”) and for reporting
whether a challenged IP then passed or failed the challenge.
Baskerville client
The machine lead anomaly prediction clearinghouse – Baskerville – is an innovative infrastructure that has been working in production on Deflect for over a year. It is a complicated set-up reliant on edge servers reporting logs to the clearinghouse, where the pre-processing for feature extraction (looking for anomalous behavior in web logs) creates vectors which are then run through the learning model. An anomaly prediction is generated and communicated back to the network edge. The clearinghouse runs on a Kubernetes cluster and requires a large amount of resources for processing.
Recently, we have split the software base into two components – the clearinghouse and the client software (operating on any Linux+nginx web server). The idea was to allow third-party clients, not using Deflect, to benefit from the clearginhouse’s predictions and the Banjax mitigation tool. In this new model, the Baskerville client is installed independently of Deflect and performs:
- Processes nginx web server logs and calculates statistical features.
- Sends features to a clearing house instance of Baskerville.
- Receives predictions from a clearing house for every IP.
- Issues challenge commands for every malicious IP in a separate Kafka topic.
- Monitors attacks in Grafana dashboards.
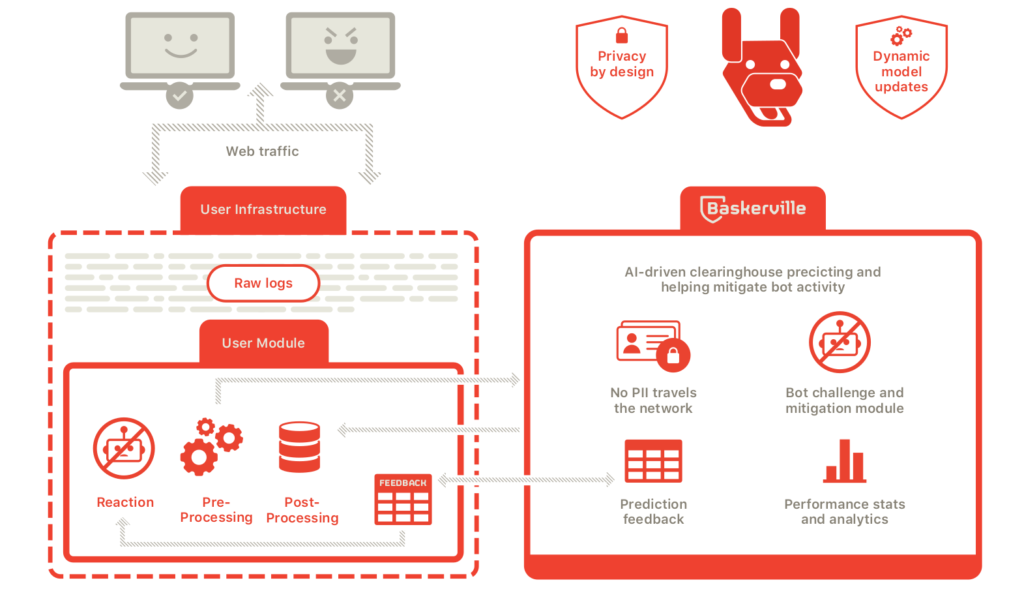
Deflect-next open source components
- Deflect – all necessary components to set up your network controller and edge servers – essentially acting as a reverse proxy to you or your clients’ origin web servers.
- Deflect-API – an interface to Deflect components
- Edgemanage – a tool for managing the HTTP availability of a cluster of web servers via DNS. If a machine is found to be under-performing, it is replaced by a new host to ensure maximum network availability.
- Banjax – basic rate-limiting on incoming requests according to a configurable set of regex patterns.
- Baskerville – an analytics engine that leverages machine learning to distinguish between normal and abnormal web traffic behavior. Used in concert with Banjax for challenging and banning IPs that breach an operator defined threshold.
- Baskerville client – edge software for pre-processing behaivoural features from web logs and communicating with the Baskerville clearinghouse for anomaly predictions.
- Baskerville dashboard – A dashboard for users running the Baskerville Client software offering setup, labeling behavior and communicating feedback to the clearinghouse
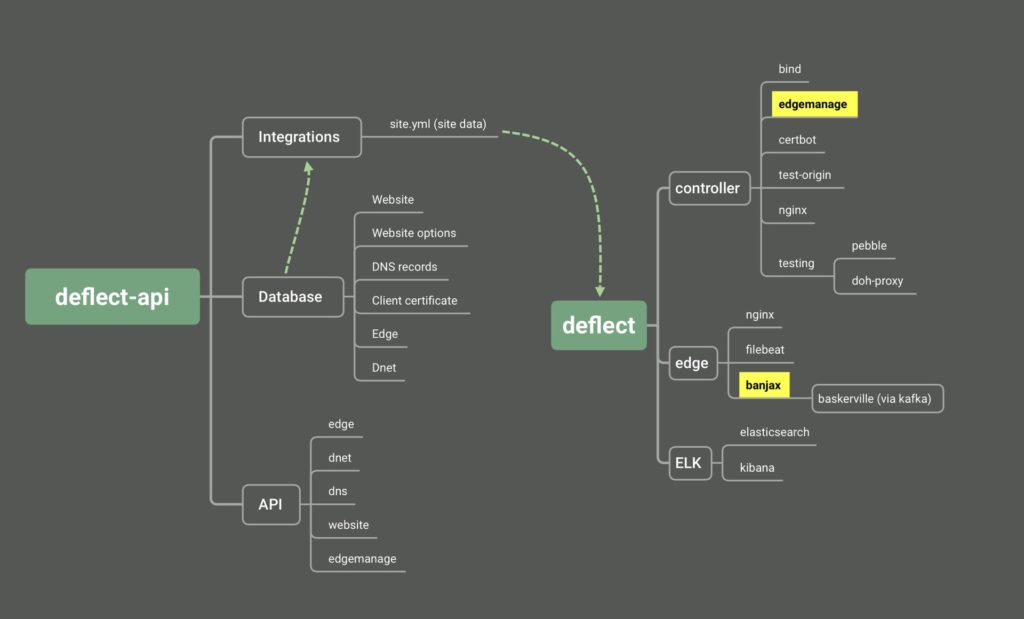